If you have been following developments in Earth observation for land-use analysis in recent years, you would know there is a lot of excitement nowadays. Some remote-sensing professionals call it the best time ever. Starting with the United States Geological Survey’s (USGS) provision of the Landsat imagery archive in 2008 and going to the development of Google Earth, cloud computing, very high-resolution imagery, radar images and others, there is so much to work with these days. Combine that with the new developments in data processing and storage, and in artificial intelligence for classification, and you can see there is an order-of-magnitude change in capacity.
Yet a challenge remains to bring these advances to professionals on the ground. How can we scale up and broadly use all these new developments? SERVIR focuses on this challenge for their five hubs around the world. Towards this effort, the program sponsors and takes part in capacity building activities that can help us achieve broad use of Earth observation technology to address sustainable development problems. Land-use and ecosystem management is one of the most important services areas for SERVIR-Amazonia, the program’s newest hub. Last week SERVIR-Amazonia participated in a workshop sponsored by SilvaCarbon and SERVIR aimed at training over 40 academic and research partners in state-of-the-art land cover change methods.
Participants in the State-of-the-Art Land Cover Change Methods workshop held in Huntsville, Alabama, USA on 20-23 August 2019.
The program started with Google Earth Engine 101. Noel Gorelick, co-developer of the platform, showed participants how to run the most basic scripts on the system, including how to display Landsat images, how to create normalized difference vegetation indices and other basic remote-sensing operations. Next, a demonstration of the SEPAL platform showed how many tools such as GEE and Collect Earth Online have been integrated into a single interface for ease of analysis.
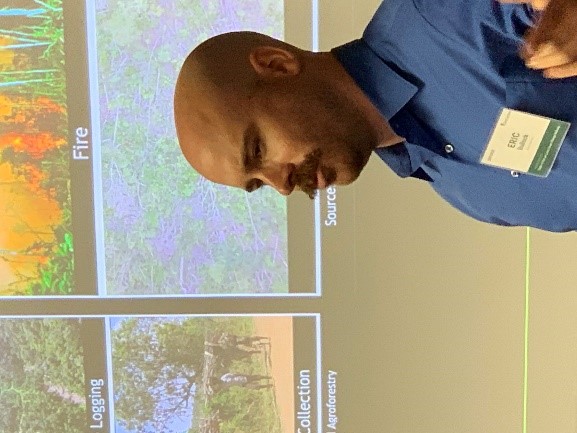
Eric Bullock of Boston University describes the Continuous Degradation Detection (CODED) algorithm for Google Earth Engine
The core of this land-use change methods workshop included several land-use change analysis applications and how they have been implemented in GEE. LandTrendr facilitates exploration of land-use change trends at the pixel and wider level, drawing on the massive Landsat archive available to the platform. The algorithm calculates common remote-sensing indices to get a sense of whether vegetation biomass is increasing or decreasing. The algorithms developers implemented LandTrendr in GEE to provide an easy way for analysts to explore vegetation trends over time. Likewise, the continuous change detection and classification (CCDC) algorithm analyzes Landsat time series data, differentiating between seasonal changes in vegetation and longer-term trends showing a change in land use. Boston University’s Eric Bullock and colleagues further advanced that work through implementation of the CODED algorithm, which estimates forest degradation from Landsat time series data. Land-use change analysis tools have also been integrated into SERVIR’s Regional Land Cover Monitoring System (RLCMS), which facilitates land-use mapping across broad areas with a yearly time step. The workshop also included state-of-the-art methods for accuracy assessment of land-use change analysis. All of these applications have been integrated into GEE, providing professionals with a powerful platform for carrying out land-use change analysis.
The workshop included a number of other initiatives aimed at improving land-use change analysis. SERVIR recently published the Synthetic Aperture Radar Handbook, a compendium of methods and tools for bringing radar data to land-use analysis. Radar remote-sensing can improve land-use change analysis in the Amazon and other tropical regions because it can penetrate clouds, show vegetation structure and detect surface water. New developments in radar remote-sensing will be used to improve deforestation monitoring systems such as Terra I, another system they showed in the workshop. Workshop instructors also demonstrated TensorFlow, a method that brings deep learning into the broader toolbox of artificial intelligence methods. Many of the different methods described above have been integrated into FAO’s SEPAL platform, which puts many of these tools into the same user interface.
The event held last week in Huntsville demonstrated why so many people are excited about all the new possibilities for improved land-use change analysis. Going forward SilvaCarbon and SERVIR together can realize the great potential for bringing these analysis methods to sustainable development problems in the Amazon, Latin America and beyond.
The International Center for Tropical Agriculture’s (CIAT) Paula Paz interprets change detection as measure
by Sentinel 1 radar imagery.
Author of this blog
Glenn Hyman